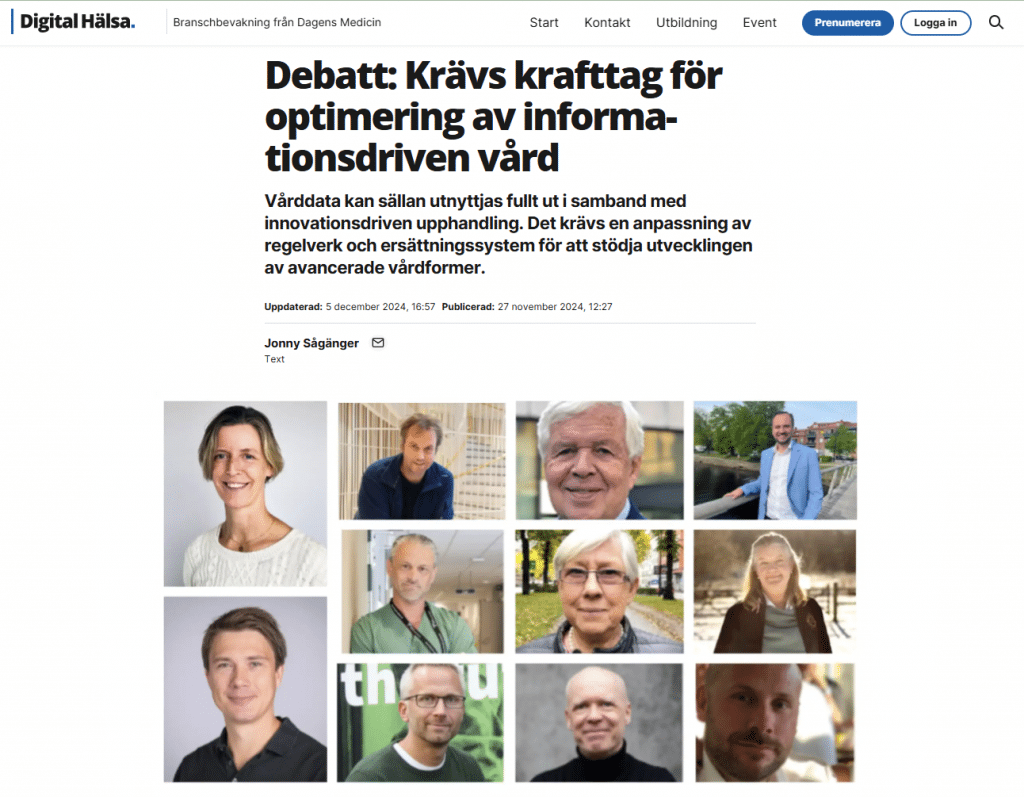
Category Archives: Life Sciences
Guest lecture at Lund University – the transformative role of AI and machine learning in drug discovery
The guest lecture at Lund University focused on the transformative role of AI and machine learning in drug discovery, with a particular emphasis on the AlphaFold model. The lecture began by discussing the importance of AI and machine learning in the drug discovery and development process, highlighting how these technologies are revolutionizing the field by enabling more efficient and accurate predictions of molecular structures and interactions.
AlphaFold, an advanced AI model developed by DeepMind. The model’s capabilities were demonstrated, showing how AlphaFold has dramatically improved the accuracy of protein structure predictions, which is crucial for understanding biological processes and developing new drugs. The impact of AlphaFold on accelerating drug discovery was emphasized, as it provides high-quality structural data that can be used to identify potential drug targets and design effective therapies.
The broader implications of AI and machine learning in the pharmaceutical industry were also discussed, including their potential to reduce the time and cost associated with drug development. Examples of successful AI-driven projects were shared, encouraging students to explore the possibilities of these technologies in their future careers.
Overall, the lecture provided valuable insights into the cutting-edge applications of AI and machine learning in drug discovery, inspiring the next generation of researchers and professionals in the field.
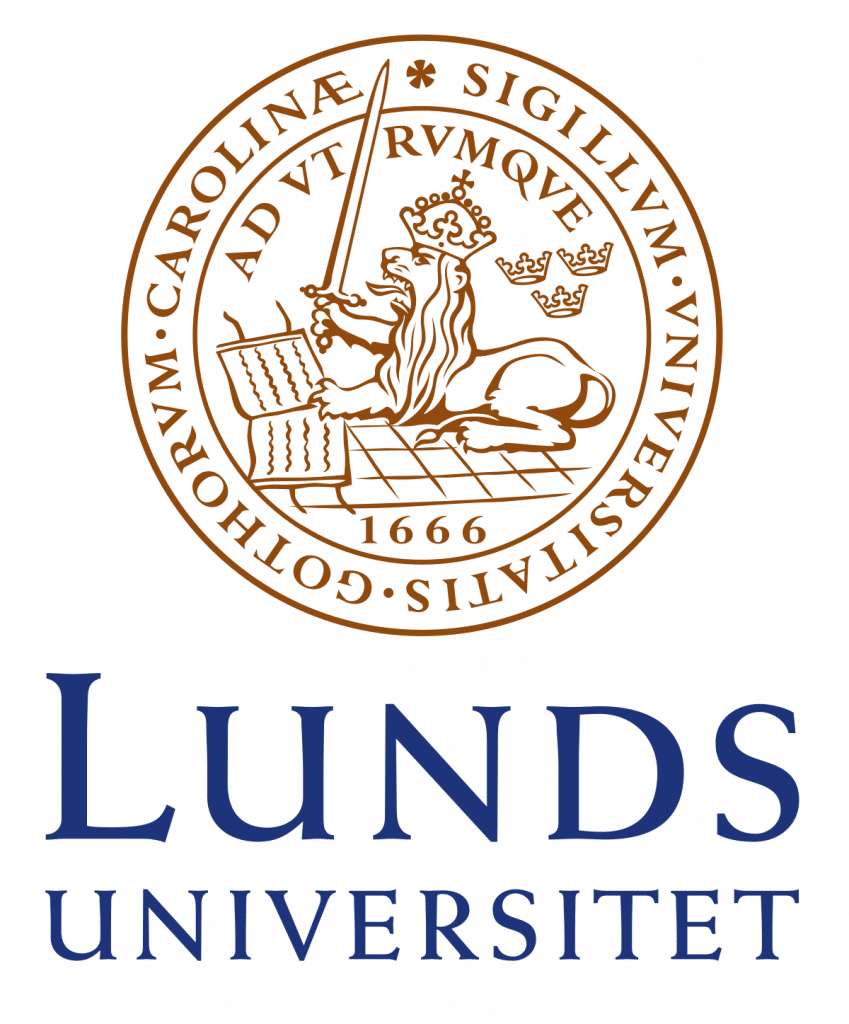
Quantum computing – what’s coming…
How we address and discuss quantum computing covers a range of important topics, reflecting the current state and future potential of this transformative technology.
One of the primary topics is the progress in developing quantum-resistant public-key cryptographic standards. Emphasizing the importance of migrating to post-quantum algorithms to ensure data security in the face of advancing quantum capabilities. Delving into the disruptive potential of quantum computing across various industries, with a particular focus on its applications in the pharmaceutical sector.
Another key area is the need for building internal expertise to differentiate between hype and genuine progress in quantum computing. Sharing learnings from working with this nascent technology is crucial for fostering a deeper understanding and practical application. Underscoring the significance of collaboration between academia, industry, and government to drive innovation and addressing the challenges associated with quantum computing.
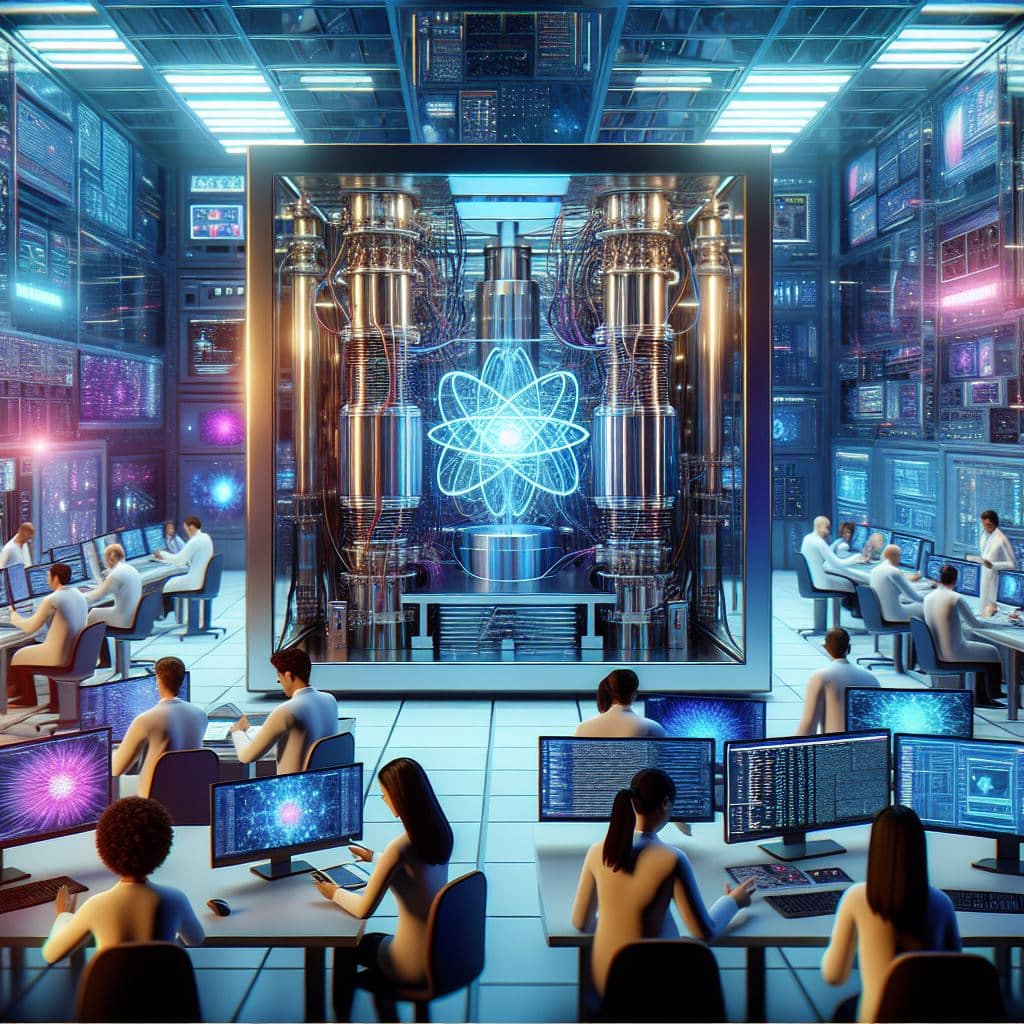
Compute clusters and energy consumption in a regulated environment
The topic “Compute clusters and energy consumption in a regulated environment” focuses on the implementation and management of high-performance computing systems, specifically those utilizing GPUs, to support advanced AI workloads. These systems are particularly relevant in regulated environments such as life sciences, and here addressed in settings where energy consumption and sustainability are critical concerns.
Compute clusters are composed of multiple nodes, each equipped with powerful GPUs, designed to deliver exceptional computational performance. The architecture of a compute cluster includes scalable units that can be configured to meet the specific needs of different applications. Each unit consists of several GPU systems, interconnected to ensure high-speed data transfer and efficient resource utilization.
One of the key challenges in deploying compute clusters in regulated environments is managing energy consumption. These systems require significant power to operate, and their energy efficiency is a critical factor in their overall sustainability. The design of compute clusters includes features such as direct liquid cooling and the use of renewable energy sources to minimize their environmental impact. Additionally, the reuse of waste heat generated by these systems for residential heating or energy storage further enhances their sustainability.
In a regulated environment, it is essential to ensure that the deployment and operation of compute clusters comply with relevant regulations and standards. This includes adhering to guidelines on energy efficiency, data security, and environmental impact. The governance and control over the assets, as well as the delegation of authority and budget management, are crucial aspects of managing compute clusters in such settings.
Overall, the implementation of compute clusters in regulated environments requires careful planning and consideration of energy consumption, sustainability, and compliance with regulatory standards. By leveraging advanced technologies and innovative solutions, organizations can harness the power of compute clusters while minimizing their environmental footprint and ensuring regulatory compliance.
Data centers of the future – LLMs for everyone
Data centers of the future – LLMs for everyone – focusing on the advancements and future prospects of data centers, in the context of supporting large language models (LLMs) and making them accessible to a broader audience. The topic explores how data centers are evolving to handle the increasing computational demands of LLMs, which are essential for various AI applications, especially in life sciences.
Key aspects of this topic include:
Infrastructure Enhancements: The need for robust and scalable infrastructure to support the training and deployment of LLMs. This involves advancements in hardware, such as GPUs and TPUs, as well as improvements in data storage and networking capabilities.
Energy Efficiency: Addressing the energy consumption challenges associated with running large-scale data centers. This includes exploring sustainable energy sources and optimizing energy usage to reduce the environmental impact.
Accessibility and Democratization: Making LLMs accessible to a wider range of users and organizations. This involves developing user-friendly interfaces, providing cloud-based solutions, and offering affordable access to powerful AI tools.
Security and Privacy: Ensuring the security and privacy of data processed by LLMs. This includes implementing robust data protection measures and adhering to regulatory requirements to safeguard sensitive information.
Innovation and Collaboration: Encouraging collaboration between industry, academia, and government to drive innovation in data center technologies and AI applications. This involves sharing best practices, conducting joint research, and fostering an ecosystem of innovation.
Overall, the topic highlights the importance of evolving data centers to meet the growing demands of AI technologies and making these advancements accessible to everyone.
Quantum Computing in Life Sciences
Quantum computing is an emerging technology that promises to revolutionize various industries by solving complex problems that are currently beyond the reach of classical computers. The initiative “Quantum Computing – the future is here, how do we use it?!” explores how we can harness this powerful technology for practical applications.
Quantum computing operates on the principles of quantum mechanics, using quantum bits or qubits, which can represent both 0 and 1 simultaneously through a phenomenon known as superposition. This allows quantum computers to process a vast number of possibilities at once, making them exceptionally powerful for certain types of computations.
One of the key areas where quantum computing is expected to have a significant impact is in drug discovery and development. By simulating molecular interactions at a quantum level, researchers can identify potential drug candidates more quickly and accurately than with classical methods. This could lead to faster development of new medications and treatments.
Another promising application is in materials science. Quantum computers can model the properties of new materials at an atomic level, enabling the design of materials with specific characteristics for use in various industries, from electronics to renewable energy.
Quantum computing also holds potential for optimizing complex systems, such as supply chains and financial portfolios. By analyzing vast amounts of data and exploring numerous scenarios simultaneously, quantum algorithms can identify optimal solutions that would be infeasible for classical computers to compute.
Despite its potential, there are still significant challenges to overcome before quantum computing can be widely adopted. These include developing stable and scalable qubit systems, error correction methods, and practical quantum algorithms. However, ongoing research and investment in this field are rapidly advancing our understanding and capabilities.
In summary, quantum computing is poised to transform various sectors by providing unprecedented computational power. As we continue to develop and refine this technology, it will be crucial to explore and implement practical applications that can harness its full potential for innovation and growth.
If you have any specific questions or need more details on any of these points, feel free to reach out!
How healthcare and AI merge together
In the healthcare sector, generative AI and other AI technologies are poised to bring transformative changes in several key areas:
Personalized Medicine: Generative AI can analyse vast amounts of patient data, including genetic information, to create personalized treatment plans. This approach can lead to more effective therapies tailored to individual patients’ needs.
Drug Discovery and Development: AI technologies can significantly speed up the drug discovery process by predicting how different compounds will interact with biological targets. Generative AI can design new molecules with desired properties, reducing the time and cost associated with bringing new drugs to market
Medical Imaging and Diagnostics: AI can enhance the accuracy of medical imaging by identifying patterns and anomalies that may be missed by human eyes. Generative AI can also create synthetic medical images to train diagnostic models, improving their performance and reliability
Virtual Health Assistants: AI-powered chatbots and virtual assistants can provide patients with instant access to medical information, answer health-related questions, and even assist in managing chronic conditions. These tools can improve patient engagement and reduce the burden on healthcare providers
Operational Efficiency: AI can optimize administrative tasks, such as scheduling, billing, and resource allocation, allowing healthcare professionals to focus more on patient care. Generative AI can also automate the creation of clinical documentation, saving time and reducing errors
Predictive Analytics: AI technologies can analyse historical data to predict future health trends and outcomes. This capability can help healthcare providers anticipate and prevent potential health issues, leading to better patient outcomes and more efficient use of resources
Overall, the integration of generative AI and other AI technologies in healthcare holds the promise of revolutionizing the industry by enhancing patient care, accelerating research and development, and improving operational efficiency.