NVIDIA’s computational capabilities are revolutionizing the biotech sector, providing unprecedented power and efficiency for various applications. In a biotech setting, NVIDIA’s supercomputers, such as the “super-pod” capabilities, are being utilized to accelerate drug discovery and development. For instance, Bristol-Myers Squibb (BMS) has an NVIDIA super-pod capability and is conducting an AI co-lab with Vant AI to accelerate Molecular Glue Drug Discovery as small molecule therapeutics.
One notable example is the collaboration between the Novo Nordisk Foundation and NVIDIA to launch a visionary AI research center in Denmark. This center, funded by a $100 million investment, aims to elevate Denmark’s researchers and innovators to the next level by leveraging one of the world’s most powerful AI supercomputers. Additionally, NVIDIA’s Tokyo-1 supercomputer is being used by leading Japanese pharmaceutical companies to accelerate drug discovery, with plans to make it accessible to medical-device companies and startups.
The future of Large Language Models (LLMs) in the biotech sector is incredibly promising. NVIDIA has introduced the “NVIDIA AI Foundations” suite of cloud services, which includes the NVIDIA NeMo language service and the NVIDIA Picasso image, video, and 3D service. These solutions enable businesses to build custom generative AI applications for various use cases, such as intelligent chat, customer assistance, professional content creation, and digital simulation3. By utilizing these services, biotech companies can develop tailored LLMs and generative AI models to enhance their research and development processes.
Generative AI is set to become a cornerstone in drug discovery and design, offering unprecedented efficiency and innovation. For example, AI tools can sift through complex biological data to identify potential biomarkers for diseases, aiding in the development of targeted therapies4. Additionally, generative AI can help identify patient subgroups most likely to benefit from a new drug, leading to more effective and personalized clinical trials.
Overall, NVIDIA’s computational capabilities and the future of LLMs in the biotech sector are poised to drive significant advancements in drug discovery, personalized medicine, and overall healthcare innovation. By leveraging these technologies, biotech companies can accelerate their research and development efforts, ultimately improving patient outcomes and transforming the healthcare landscape.
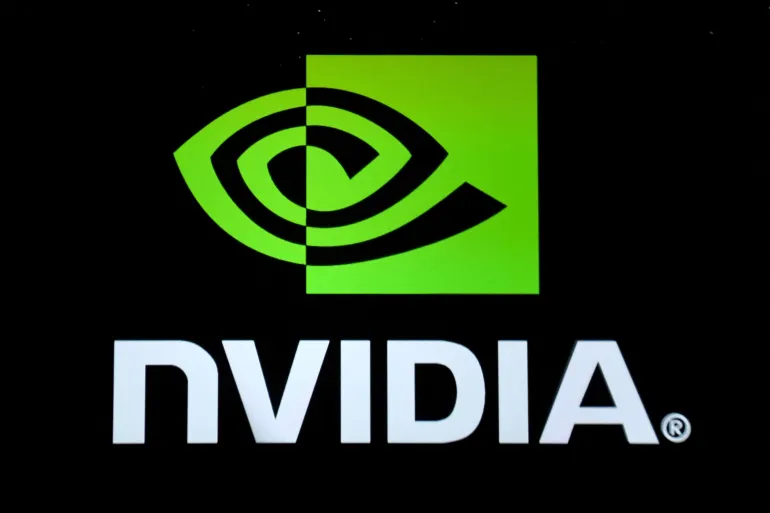